SBYC Holiday CHRF 2019 on J70 Escape
A small warning, some salty language.
A fun day on the ocean. We had wind gusts around 18 with average winds of 15vmph plus it was COLD. The wind direction was unusual. We still had an angle to fly the kite.
We sailed course HD7 reverse (C/H/K/Finish) for 5.5 nautical miles (6.3 miles).
Our club handicap for the day was still bad. CHRF racing is like golfing handicaps. It changes from race to race.
Speed Stats in MPH during the race: Min. 1st Qu. Median Mean 3rd Qu. Max. 2.311 7.163 7.729 7.852 8.326 14.017
Happy Thanksgiving 2019
Happy Thanksgiving... 🇹🇷 🍾 🍽
🍰
Happy Thanksgiving! 🦃 pic.twitter.com/52ojfhjYj2
— PEANUTS (@Snoopy) November 28, 2019
Today of strava firsts
Talk about first for me. Ran over a half marathon (13.1 miles), got my climbing badge, and am 0.1 km under 200km goal.
I am not a young thing. I could not do this when I was in best of shape. In 2016 when I decided that I was fat, and I was going to lose weight to be healthy walking a few miles a day was an accomplishment.
I have some fanticy accomplishements that I wan to do: 5K, 10K, half marathon, Triautholon. You know what? They do not seem too inimidating like they did in 2016. I just need to sign up and participate.
It is funny to me, but the badges on strava do have meaning. You do not get them for showing up, they are earned by doing.
Twitter and the Cave Fire
Twitter has been a good source of information in realtime outside the pornbots and climate change virtue signalers (time and place appropriateness).
Tweet #CaveFireCave Fire
Yesterday around 4:30pm, I got this text:
Fire dept enroute for Fire in the Highway 154 area on the Santa Barbara Side.
When I got out of work I took this picture from SBCC East Campus around 5pm November 25.
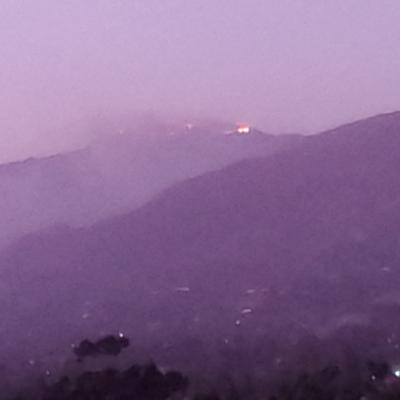
By 7pm ish I took this at 2837 De La Vina St Santa Barbara, CA
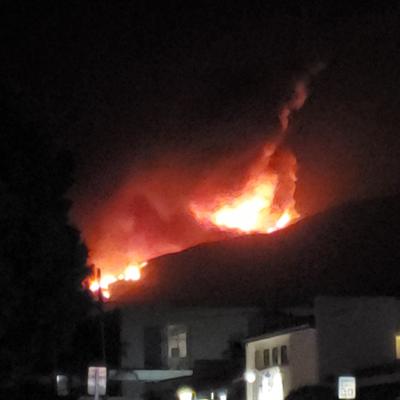
I have a friend who lives in the area. I am glad she is more prepared than I am and high tailed it. Her house is not in danger, but Santa Barbara is really just one bad wind change away from being burned down.
#CaveFire - A Los Angeles County Firehawk copter makes a water drop near a home off Cieneguitas Rd in Santa Barbara Monday night. pic.twitter.com/LrzWUsm6A7
— SBCFireInfo (@EliasonMike) November 26, 2019
The air was clean this morning, but I can smell the fire now. Time to find the N95 masks that I bought from the last fire.
Now for the editorial. We have a good airport. However, fire fighting aircraft is located in Santa Maria and Los Angeles. I am thankfult to Los Angeles for sharing their resources last night. Fires like this get out of hand because of lack of quick response. Santa Barbara USED to have fire fighting aircraft. There is a part of me that believes that we would have such aircraft if it were important to our representatives.
A note: I think growing up in in Santa Barbara, I am remeber, how fast response was. Even with resources close, fires could get out of hand. This fire went from a few acres to over 3,000 in hours. Our fire department was on top of it. A single helecopter around three hours later. Major air assets around 16 hours later. It was scarry last night because there was gale warnings (34 to 47 knots) and wind direction could have swepted the fire through town.
I am going to say this, I am appreciative of the effort to get the fire under control. It is first rate.
Resources involved in responding to the #cavefire include:
— Santa Barbara County (@countyofsb) November 26, 2019
600 firefighters
5 bulldozers
9 helicopters
10 fixed wing aircraft, and more
Thank you to all first responders and to all the crews in the region who responded to assist #SantaBarbaraCounty
Lifestyle changes to prevent Alzheiner's
Just read A New Treatment for Alzheimer's? It Starts With Lifestyle by Linda Marsa.
I feel that we should all read it. Partially so that we can better take care of our love ones and take better care of our selves. I don't know if it is correct or not, however, to me it makes a lot of sense.
It talks about bad actors. Bad actors include things that increase risk (probably noninclusive):
chronic stress
lack of exercise
lack of restorative sleep
toxins from molds
fat-laden fast foods
too much sugar
being pre-diabetic
Plus
sedentary lifestyles
poor eating habits
Type 2 diabetes
insulin resistance
skyrocketing obesity (body positivity or it is ok to be medically obese ??)
Summary protocal to combat the problem:
Optimizing sleep and getting at least eight hours of shut-eye every night.
Fasting at least 12 hours a day; patients usually don’t eat anything after 7 p.m. until the next morning.
Frequent yoga and meditation sessions to relieve stress.
Aerobic exercise for 30 to 60 minutes, at least five times a week.
Brain training exercises for 30 minutes, three times a week.
Eating a mostly plant-based diet: broccoli, cauliflower, Brussels sprouts, leafy green vegetables (kale, spinach, lettuce).
Cutting out high-mercury fish: tuna, shark and swordfish.
Drinking plenty of water.
Eliminating gluten and sugars. Cutting out simple carbs (bread, pasta, rice, cookies, cakes, candy, sodas).
This is just a short summary. I hope you read the full article. I plan to add more to this post, just that right now I other responcibilites are calling. I hope to update later.
2019 Trending Value Stocks as up November 7th
In the beginning of the year, I had created a real money port using O'Shaughnessy Trending value. I am a big fan of his What Works in Wallstreet book. In August I had liquidated the possion and just replaced it with the current Small Dogs of the Dow. The Small Dogs of the Dow are not as good as just buy the ETF SPY which is my bench mark. But it is good.
Part of the reason why I am doing this post is that I am starting to feel that I could have purchased SPY when I started investing again in late 1999, made more money and had less headaches by just buying SPY and let it ride. The Market is smarter than I am. It is more for my Investment journey since I feel that I need to be more conservative in my choices.
This post compares three choices that I was thinking about January 1, 2019: Dogs of the Dow, Trending Value, SPY. I had bet very wrong with Trending Value.
The Trending Value stocks were generated using AAII Stock Investor Pro.
#Load Libaries
pacman::p_load("quantmod", "tseries", "PerformanceAnalytics")
Trending Value Stocks that I purchase. Barns & Noble is commented out since it was purchased by Elliott June 7th Removing is oversimplification but still gives me a picture of performance.
trendvalueSymbols <-
c(
"AFL",
"AGO",
"ANAT",
# "BKS", # Purchased
"CHA",
"CHL",
"CLW",
"CSIQ",
"CTB",
"EIG",
"ELP",
"GHC",
"HRB",
"KEN",
"KT",
"NRP",
"OFG",
"PDLI",
"REGI",
"SBS",
"SCVL",
"SIM",
"SKM",
"UAL",
"VIV"
)
Dogs of the Dow for 2019
DoDSymbols <-
c("IBM", "XOM", "VZ", "CVX", "PFE", "KO", "JPM", "PG", "CSCO", "MRK")
Benchmark: SPY
SpyderSymbols <- c("SPY")
Get Stock Data
options("getSymbols.warning4.0"=FALSE)
getSymbols(trendvalueSymbols,
src = 'yahoo',
from = '2019-01-08',
to = '2019-11-08')
getSymbols(SpyderSymbols,
src = 'yahoo',
from = '2019-01-08',
to = '2019-11-08')
getSymbols(DoDSymbols,
src = 'yahoo',
from = '2019-01-08',
to = '2019-11-08')
Put data in lists
pricesTV <- list()
pricesSPY <- list()
pricesDoD <- list()
for (i in 1:length(trendvalueSymbols)) {
pricesTV[[i]] <- Ad(get(trendvalueSymbols[i]))
}
pricesTV <- do.call(cbind, pricesTV)
colnames(pricesTV) <- c(trendvalueSymbols)
for (i in 1:length(SpyderSymbols)) {
pricesSPY[[i]] <- Ad(get(SpyderSymbols[i]))
}
pricesSPY <- do.call(cbind, pricesSPY)
colnames(pricesSPY) <- c(SpyderSymbols)
for (i in 1:length(DoDSymbols)) {
pricesDoD[[i]] <- Ad(get(DoDSymbols[i]))
}
pricesDoD <- do.call(cbind, pricesDoD)
colnames(pricesDoD) <- c(DoDSymbols)
Generate Returns and prep for charting
# generate daily returns
returnsTV <- na.omit(ROC(pricesTV, 1, "discrete"))
returnsSPY <- na.omit(ROC(pricesSPY, 1, "discrete"))
returnsDoD <- na.omit(ROC(pricesDoD, 1, "discrete"))
#Prep for charting
portfolio.tv <-
Return.portfolio(returnsTV, wealth.index = TRUE, verbose = TRUE)
portfolio.spy <-
Return.portfolio(returnsSPY, wealth.index = TRUE, verbose = TRUE)
portfolio.dod <-
Return.portfolio(returnsDoD, wealth.index = TRUE, verbose = TRUE)
portfolios.2 <-
cbind(portfolio.tv$returns,
portfolio.spy$returns,
portfolio.dod$returns)
colnames(portfolios.2) <-
c("Trending Value", "SPYders", "Dogs of Dow")
Chart
chart.CumReturns(
portfolios.2,
wealth.index = TRUE,
legend.loc = "bottomright",
main = "Growth of $1 investment",
ylab = "$"
)
Returns
table.AnnualizedReturns(portfolios.2)
I don't have time to go into detail this morning. My day job calls. However the difference in returns is glaring. I will let you draw your own conclusions on this.
Jupyter test with R kernel
Just wanted to see if I could do a post with Nikola using an R kernel with Jupyter.
This is the test code from: https://docs.anaconda.com/anaconda/navigator/tutorials/r-lang/
library(dplyr)
iris
library(ggplot2)
ggplot(data=iris, aes(x=Sepal.Length, y=Sepal.Width, color=Species)) + geom_point(size=3)
November 2019 pull-up challange
Well, I failed my October 2019 Pullup Challange. Going to try again in November to get up to 10 pullups a day by the end of the month.
November schedule:
Date |
# of Pullups |
---|---|
11/1/19 |
1 |
11/2/19 |
1 |
11/3/19 |
2 |
11/4/19 |
2 |
11/5/19 |
2 |
11/6/19 |
3 |
11/7/19 |
3 |
11/8/19 |
3 |
11/9/19 |
4 |
11/10/19 |
4 |
11/11/19 |
4 |
11/13/19 |
5 |
11/14/19 |
5 |
11/15/19 |
6 |
11/16/19 |
6 |
11/17/19 |
6 |
11/18/19 |
7 |
11/19/19 |
7 |
11/20/19 |
7 |
11/21/19 |
8 |
11/22/19 |
8 |
11/23/19 |
8 |
11/24/19 |
9 |
11/25/19 |
9 |
11/26/19 |
9 |
11/27/19 |
10 |
11/28/19 |
10 |
11/29/19 |
10 |